Hierarchical Machine Learning
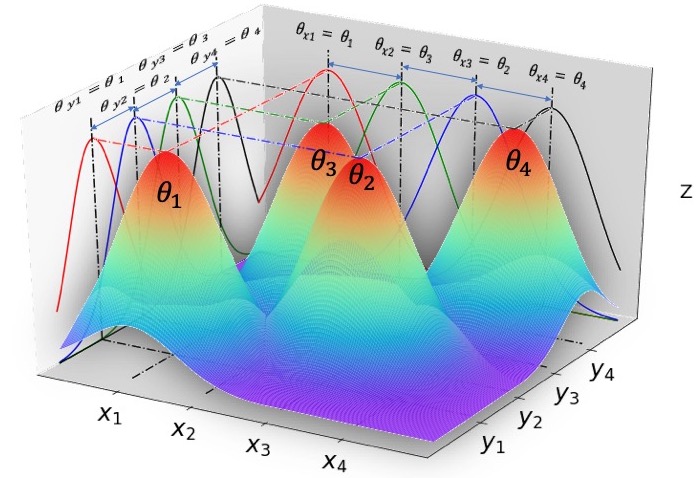
In this research we proposes a hierarchical learning strategy for generation of sparse representations which capture the information content in large datasets and act as a model. The hierarchy arises from the approximation spaces considered at successively finer data dependent scales. We present a detailed analysis of stability, convergence and behavior of error functionals associated with the approximations and well chosen set of applications. Results show the performance of the approach as a data reduction mechanism on both synthetic (univariate and multivariate) and real datasets (geo-spatial, computer vision and numerical model outcomes). The sparse model generated is shown to efficiently reconstruct data and minimize error in prediction.